MMM vs. MTA: Which attribution model should you choose?
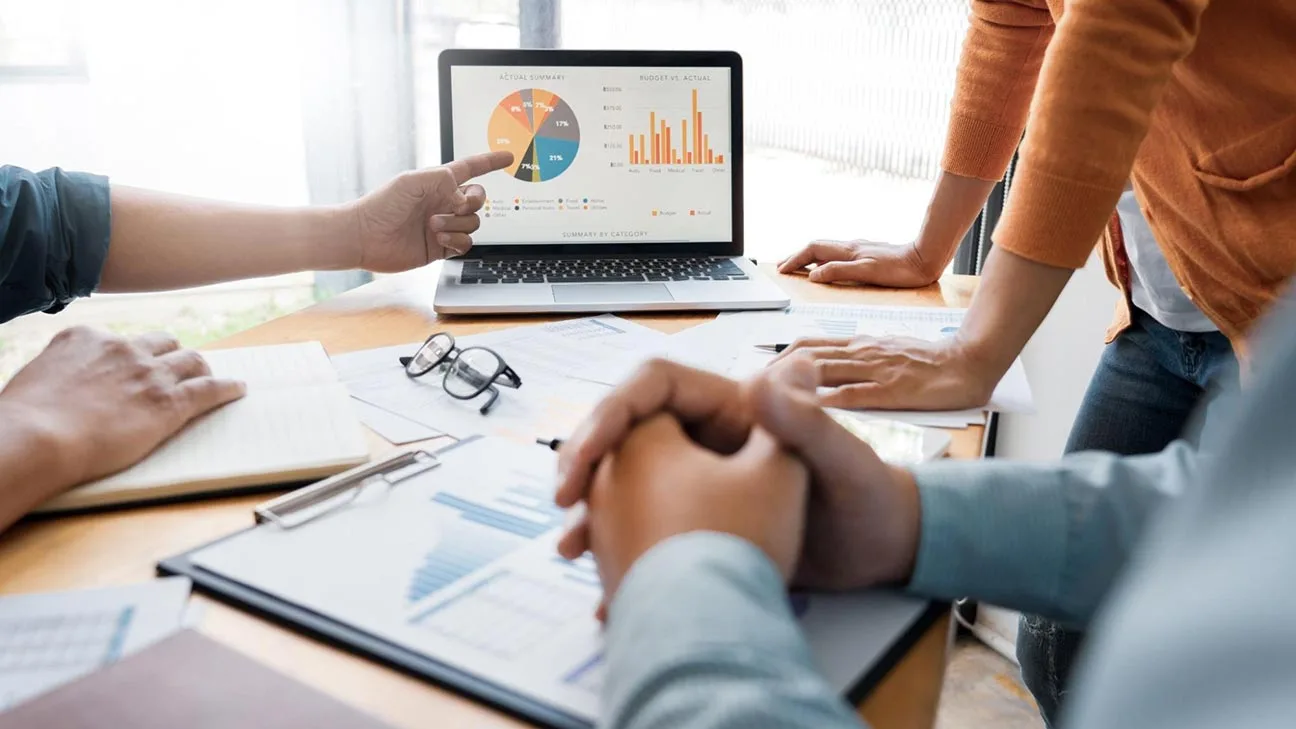
As marketers, it can be challenging to figure out which marketing channels, metrics, customer touch points, and engagement strategies really matter when there’s so much data to sift through. It’s easy to feel overwhelmed and uncertain of how to pick and allocate resources in the right direction for maximum impact. This is where the ongoing debate of marketing mix modeling (MMM) vs. multi-touch attribution (MTA) highlights a critical question: How do you choose an attribution model that maximizes your investments while positioning your enterprise for sustainable growth?
The answer lies in understanding the strengths and drawbacks of each model and how they align with your specific marketing goals. This blog will break down the fundamentals of MMM vs. MTA and help guide you in choosing the right attribution modeling approach.
What is marketing mix modeling?
Marketing mix modeling, also known as media mix modeling, is a top-down approach to marketing measurement that uses statistical analysis to quantify the impact of various marketing channels and tactics on sales and other key performance indicators (KPIs). It typically relies on aggregate data to estimate the impact of each marketing input on your overall business outcomes.
How does MMM work?
Most MMM models use regression analysis—a statistical analysis method—to understand the relationship between the following:
- A dependent variable: The outcome you want to predict or explain, like sales
- One or more independent variables: The factors you believe will influence the outcome, like marketing spend, price, or competitor activity
Think of it as trying to figure out how different ingredients (independent variables) impact the taste of a cake (dependent variable). By analyzing historical data, you can build a model that tells you how each independent variable affects the final result.
For example, the model might find that a 10% increase in social media advertising spend leads to a 3% increase in sales, while a 10% increase in price results in a 5% decrease in sales. These insights help marketers make informed decisions about budget allocation and optimization.
Why would you choose MMM?
Here are some of the top reasons why some businesses choose MMM.
A holistic view of marketing performance
MMM provides a broad view of how different marketing channels and tactics perform. By analyzing both online and offline channels, it offers insights into the overall effectiveness of various marketing strategies. This helps in understanding the synergy between different marketing efforts.
Identification of long-term trends
MMM is valuable for spotting long-term trends and understanding the historical impact of different marketing activities. This helps in predicting future performance and guiding long-term strategic planning.
Handling data limitations and privacy concerns
MMM is resilient to data limitations and privacy issues because it relies on aggregate data rather than individual-level tracking. This makes it a useful tool in environments with strict data privacy regulations.
Key considerations when employing MMM
- Granularity and individual insights: While MMM offers a comprehensive overview, it often lacks the granularity needed for customer-level insights. Integrating MMM with other methods that capture individual-level data may be necessary for more detailed analysis.
- Responsiveness to market changes: MMM relies heavily on historical data, which can make it less responsive to rapid changes in the market or emerging marketing channels. Keeping the model updated with recent data and trends is crucial for maintaining relevance.
- Resource intensity: Building and maintaining an MMM model requires substantial time and resources. It involves complex statistical work and ongoing data management. Ensuring you have the necessary resources and expertise is essential for successful implementation.
- Attribution challenges: MMM can struggle with attributing success to specific campaigns, especially in a fragmented digital landscape. Combining MMM with other attribution methods might provide a more comprehensive view of campaign effectiveness.
What is multi-touch attribution?
Multi-touch attribution tracks every interaction a customer has with your brand before they purchase. It’s a bottom-up approach to marketing measurement that assigns fractional credit to each interaction, giving you a granular understanding of how different marketing tactics and channels influence customer behavior and drive conversions.
How does MTA work?
By tracking and analyzing user-level data across multiple channels and devices, MTA creates a detailed picture of how customers interact with a brand before converting. Here’s how MTA gathers, connects, and analyzes data to help reach meaningful conclusions.
Gathering the breadcrumbs of user interactions
There are two main ways to collect data for MTA: through urchin tracking modules (UTMs) and pixels.
- UTMs: UTM parameters are added to URLs to track website visitors from specific sources. You can use UTMs for both offline and digital marketing campaigns. In the case of offline ads, you can create QR codes that lead to a landing page with a specific UTM to keep track of the offline campaigns. You can integrate this information with a marketing analytics platform like Buffer or Ahrefs to keep track of them.
- Pixels: Pixels are small pieces of code added to your website to capture essential user actions like button clicks, form submissions, and other specific page interactions.
Some platforms, like Yelp, offer pixel-tracking abilities. For instance, Yelp Pixel helps businesses gain a complete picture of how their Yelp Ads drive conversions across the entire customer journey. Once you place the Yelp pixels across your website’s landing pages, you can effortlessly track and attribute your sales to specific Yelp campaigns.
Similarly, you can use other advertising platforms’ pixel-tracking abilities to collect data for MTA models.
Connecting the dots across customer journeys
The initial data collected from different stages of the customer journey is often fragmented. Combining this data and arranging it in the right context across the entire customer journey is often challenging, especially with the rise of multi-device households.
Let’s say that a user browses for your product or service on their smartphone and then moves to their laptop to complete their purchase. It would be challenging to attribute the sales made from the laptop to the research done on the smartphone.
However, with the right tools and techniques, you can still keep track of such cross-device customer journeys. MTA employs a combination of deterministic and probabilistic matching techniques to create a unified customer view, no matter how complicated it is.
Deterministic matching relies on definitive data points like login information or email addresses to link user activity across devices. Probabilistic matching, on the other hand, uses advanced algorithms to analyze patterns in user behavior—such as device fingerprints, IP addresses, and browsing habits—to identify unique users with a high degree of accuracy.
This data helps go beyond the limitations of rule-based marketing attribution models (like first-touch or last-touch attribution) by connecting the dots across customer journeys to adapt to the unique characteristics of each customer segment and the evolving consumer behavior.
Choosing the best multi-touch attribution modeling framework
Once you have the complete data for the customer journey mapped out, all that’s left to do is choose the right MTA model type to apply.
There are different types of MTA to choose from:
- Linear attribution: This MTA type distributes credit equally among all touch points, assuming that each interaction plays an equal role in driving the conversion.
- Time-decay attribution: This attribution type assigns more credit to touch points closer to the conversion, assuming that interactions nearer to the point of sale have a greater impact on the decision to purchase.
- Position-based or U-shaped attribution: A fixed percentage of credit (e.g., 40%) is assigned to the first and last touch points, with the remaining credit (e.g., 20%) distributed equally among the middle touch points. This model assumes that the first and last interactions are more influential than the others.
- Custom data-driven attribution: Using machine learning algorithms, this MTA type analyzes patterns in historical data and assigns credit based on the actual impact of each touch point on conversion probability. This approach is more complex but can provide more accurate and actionable insights.
There are also different powerful machine learning techniques used in MTA, such as the Shapley Value algorithm. The Shapley Value, which comes from cooperative game theory, calculates the probability that each touch point has to make a conversion by considering all possible sequences of customer journeys. This approach helps to address the biases inherent in rule-based models and provides a more balanced distribution of credit.
Such elaborate MTA algorithms offer a detailed understanding of how different touch points contribute to conversions, empowering marketers to identify the most effective channels, ad placements, and creative elements for each customer segment. This helps allocate resources more efficiently toward long-term strategies that can maximize return on investment (ROI).
For instance, the enterprise-dedicated attribution team at Yelp works closely with businesses to design and recommend a custom, data-driven MTA model that accurately reflects their customer journey.
Ultimately, it’s most important to choose the most suitable attribution model based on your industry, sales cycle length, business goals, and the standard buyer’s journey.
Why would you choose MTA?
While there are numerous reasons for businesses to choose MTA, here are some of the most vital ones.
Detailed customer path analysis
MTA provides a thorough view of individual customer journeys, allowing for tactical optimizations based on how each touch point influences the conversion. This granular insight helps in understanding the role of each interaction in the overall customer experience.
Enhanced personalization
By identifying high-value touch points and customer segments, MTA enables personalized marketing strategies.
Marketers can also tailor their messaging at individual touch points based on customer segments, their interactions, and the specific stage of the customer journey, ensuring more relevant and effective communication.
Real-time decision-making
MTA offers timely insights with the ability to update data on a daily or weekly basis. This allows for agile adjustments to ongoing marketing strategies based on the most current information and responses.
Improved budget allocation
The insights gained from MTA help in more effective budget allocation by highlighting which touch points are most influential. This ensures that resources are invested in channels and tactics that deliver the highest ROI and brand recall.
Cross-channel, cross-device effectiveness
MTA integrates data from multiple digital channels and devices, providing a holistic view of marketing performance. This helps you track conversions across devices and also to understand how different channels work together to drive conversions.
Key considerations when employing MTA
- Complexity of attribution models: The variety of attribution models available can introduce complexity and subjectivity in credit allocation. Choosing the right model that aligns with your marketing objectives is crucial for accurate insights.
- Resource and expertise requirements: Implementing MTA requires substantial resources and expertise. Managing the complexities of data integration, tracking, and model selection demands advanced tools and skilled professionals.
- Restrictions on third-party data: As data privacy regulations tighten, relying on third-party data can become increasingly challenging. However, there are ways to still get sufficient data without relying on third-party cookies. For example, Yelp’s Conversions API (CAPI) helps address this by allowing you to match your first-party data with Yelp’s first-party data, broadening the scope of what you can track and analyze. Such innovative approaches allow you to still gain valuable insights while navigating data restrictions.
MMM vs. MTA: an in-depth comparison of their foundational concepts
While MMM and MTA aim to measure and optimize marketing efforts, both approach it from fundamentally different angles. Understanding these differences of MMM vs. MTA is crucial to building a comprehensive and effective measurement strategy.
The fundamental divide: top-down vs. bottom-up approach
At the heart of the MMM vs. MTA debate lies a fundamental divide between macro- and micro-level measurement. MMM takes a top-down view, using aggregate data to model the overall impact of marketing channels and tactics on business outcomes. It’s like looking at a forest from a helicopter, seeing the broad patterns and interactions between different elements.
In contrast, MTA adopts a bottom-up approach, meticulously tracking individual customer journeys and attributing value to each touch point along the path to conversion. It’s like examining the forest from the ground, looking at each tree, understanding its growth and interactions with its immediate surroundings.
This fundamental difference has far-reaching implications for data requirements, privacy concerns, and adaptability to the changing digital landscape. MMM’s aggregate approach makes it more resilient to data limitations and privacy regulations, as it doesn’t rely on individual-level tracking. However, it may miss granular insights that could drive tactical optimizations.
MTA’s micro-level view offers the promise of personalization and real-time optimization, as it can identify high-value touch points across customer segments. However, it requires extensive tracking and adhering to strict privacy regulations like GDPR and CCPA.
The data problem: breadth vs. depth
MMM thrives on broad, historical datasets, often spanning years of marketing spend, sales figures, and external factors like seasonality or economic indicators. This breadth allows MMM to capture long-term effects and account for complex market dynamics that may be invisible at the individual customer level.
Conversely, MTA demands deep, granular data on individual customer interactions across multiple touch points. It requires sophisticated tracking mechanisms and relies heavily on digital signals. This depth enables MTA to provide valuable insights into the sales funnel, potentially uncovering micro-moments that significantly influence purchase decisions.
However, this data divide also presents challenges. MMM’s reliance on aggregate data can make it less responsive to rapid market changes or emerging channels. It may struggle to capture the impact of new tactics or technologies that don’t have a long history of data.
On the other hand, with MTA, you need to establish strong cross-device tracking across both online and offline touch points, relying on tangible identifiers to stitch together customer journeys.
The attribution puzzle: assigning credit where it’s due
One of the critical questions every marketer faces is: How do we assign credit for a conversion to that specific marketing touch point?
This is where the two methodologies diverge the most.
MMM approaches attribution from a high-level perspective, using statistical modeling to estimate the contribution of each marketing channel to overall sales. It aims to answer strategic questions like:
- How much incremental revenue did our TV advertising generate last quarter?
- What is the optimal budget allocation across channels to maximize ROI?
- Which marketing channel brought in the most conversions last year?
However, MMM doesn’t provide granular credits to individual touch points on the customer journey, which can leave marketers at a disadvantage when they need to optimize or pick the right touch points to use in a campaign.
Conversely, MTA dives deep into individual customer journeys, employing various models to assign fractional credit to each touch point. It offers advanced insights into the incrementality of each touch point—measuring how each interaction contributes additional value to conversions—and how customer interactions evolve over time.
MTA aims to answer tactical questions like:
- Which touch points in this strategy seem to slow down the conversions?
- How can we optimize our bidding strategies and ad placements based on a touch point’s performance?
- Where, in this customer journey, are prospects dropping off the most?
- What is the ideal frequency and sequence of touch points to maximize the likelihood of conversions?
- How do changes in customer behavior or market conditions impact the effectiveness of each touch point over time?
Apart from these foundational questions, MTA models offer even deeper insights.
For instance, MTA can reveal how different types of touch points (e.g., social media ads vs. email campaigns) interact and compound their effects on the conversion path. It can also identify touch points that may be overlooked but have significant cumulative effects when considered across broader customer journeys. This level of detail supports refined strategies for resource allocation and enhances the precision of customer engagement tactics.
Which attribution path should you follow?
Rather than thinking of MMM vs. MTA, the most successful measurement strategies involve a convergence of these two approaches, leveraging the strengths of each to ensure the success of marketing activities. While MMM has traditionally been used for strategic budget allocation, MTA’s granular, customer-level insights have proven to be more valuable for planning and executing marketing activities across the board.
MTA’s ability to track individual customer journeys and integrate with other marketing technologies—such as personalization engines, content recommendation systems, and real-time bidding platforms—makes it an indispensable tool for day-to-day marketing execution. This adaptability ensures that marketing strategies remain aligned with current consumer behaviors and emerging trends.
While MMM remains useful for high-level channel selection and budget decisions, MTA can handle many tasks traditionally managed by MMM—like analysis of channel effectiveness, budget allocation, customer segmentation insights, and campaign performance measurement. This enables marketers to make data-driven decisions that span both strategic and tactical dimensions.
For example, MTA can help marketers determine not only which channels to invest in but also how to optimize specific tactics within those channels, such as ad placements, bidding strategies, and creative variations. This level of insight and optimization is crucial for driving measurable business impact in an increasingly complex and competitive marketing landscape.
In essence, while MMM provides valuable strategic guidance in understanding broad trends and planning marketing budgets, MTA provides detailed insights into how each touchpoint contributes to conversions. Both approaches have their unique strengths, and businesses should carefully consider their specific goals when choosing between them.
It’s also crucial to consider the features and tools available on the platforms you market and advertise to ensure they align with and support the marketing model of your choice.
For instance, Yelp helps marketers apply both MMM and MTA to their advertising campaigns. Yelp can capture and feed the data required for MMM models. In the case of MTA, Yelp offers various built-in tools to track and connect every customer interaction, like:
Also, it supports integration with third-party tools like Foursquare, InMarket, and Neustar.
Choosing the appropriate marketing approach lets you not only plan smarter but also act with pinpoint accuracy—ensuring your campaigns are both strategic and spot-on. In some cases, the integration of both MMM and MTA could be the key to unlocking the full potential of your marketing efforts.
What’s your choice of marketing model: MMM, MTA, or both?
The choice of MMM vs. MTA often hinges on the organization’s data infrastructure, customer interactions, and strategic priorities in terms of long-term planning versus tactical optimization.
While MMM is great for planning out the marketing budget and getting a bird’s eye view of the overall marketing performance, MTA’s wealth of granular touch point data, powerful modeling capabilities, and seamless integrations make it easier to glean and apply insights at scale. Both models working in tandem can facilitate a holistic approach to marketing attribution, blending strategic insights with granular touchpoint data that optimizes overall marketing performance.
Looking for ways to gather more customer-centric data to use with your MMM and MTA models? Explore these consumer research methods to truly understand what goes on in the minds of your customers—and leverage this information to finesse your marketing strategies.